LATEST POSTS
Home
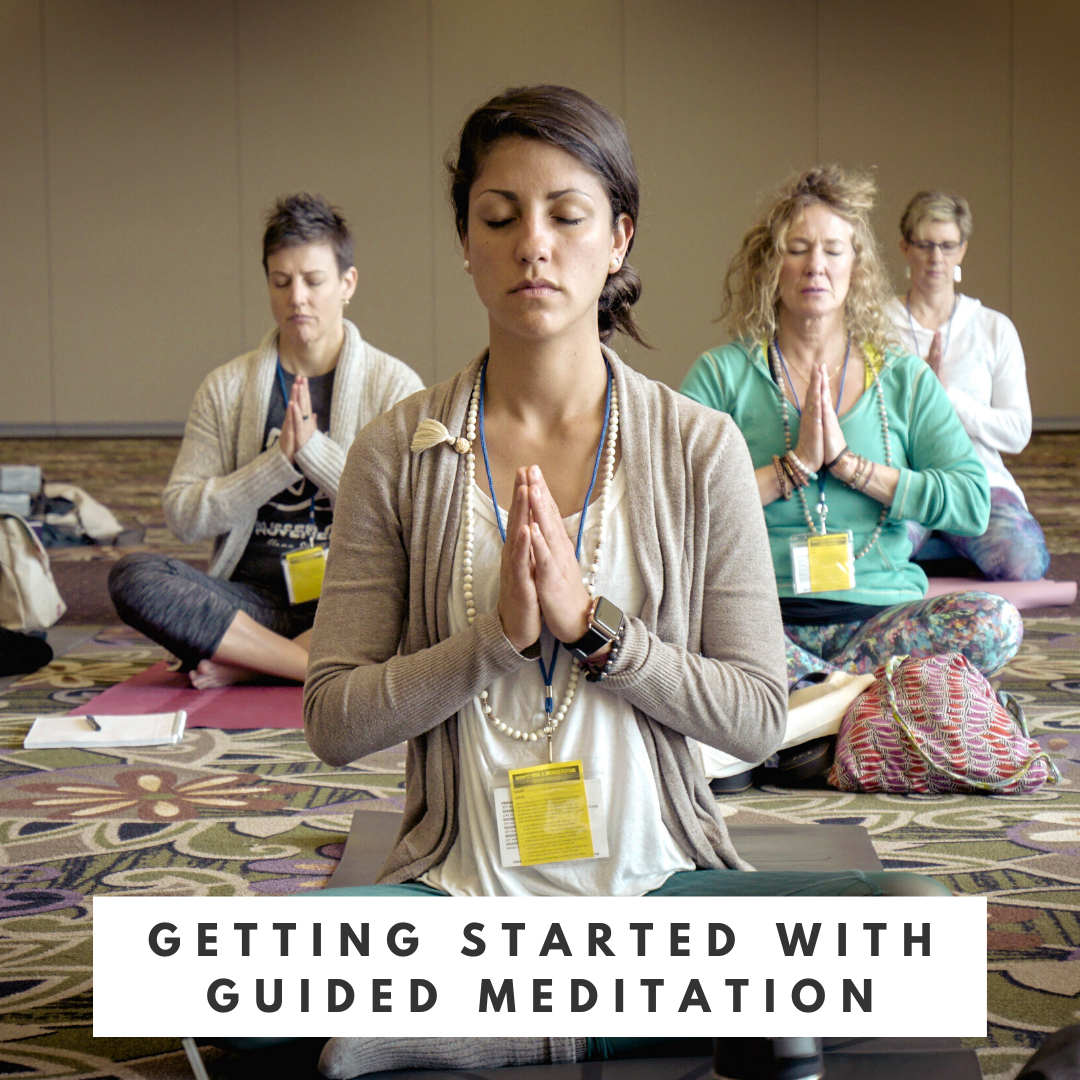
Getting started with Guided Meditation
Are you ready to thrive? Our ancient yogic wisdom techniques will help you unlock your true potential. Forget what you think you know about yoga—this is about mastering your mind, boosting your productivity, and living a life of purpose.